Original Research | ||||||||||||||||||||||||||||||
Deep Learning based Frameworks for Real-time Cyber Threat Analysis Laila Almutairi.
|
How to Cite this Article |
Pubmed Style Laila Almutairi. Deep Learning based Frameworks for Real-time Cyber Threat Analysis. Journal of Engineering and Applied Sciences. 2023; 10(2): 119-137. doi:10.5455/jeas.2023110109 Web Style Laila Almutairi. Deep Learning based Frameworks for Real-time Cyber Threat Analysis. https://jecasmu.org/?mno=180842 [Access: July 06, 2024]. doi:10.5455/jeas.2023110109 AMA (American Medical Association) Style Laila Almutairi. Deep Learning based Frameworks for Real-time Cyber Threat Analysis. Journal of Engineering and Applied Sciences. 2023; 10(2): 119-137. doi:10.5455/jeas.2023110109 Vancouver/ICMJE Style Laila Almutairi. Deep Learning based Frameworks for Real-time Cyber Threat Analysis. Journal of Engineering and Applied Sciences. (2023), [cited July 06, 2024]; 10(2): 119-137. doi:10.5455/jeas.2023110109 Harvard Style Laila Almutairi (2023) Deep Learning based Frameworks for Real-time Cyber Threat Analysis. Journal of Engineering and Applied Sciences, 10 (2), 119-137. doi:10.5455/jeas.2023110109 Turabian Style Laila Almutairi. 2023. Deep Learning based Frameworks for Real-time Cyber Threat Analysis. Journal of Engineering and Applied Sciences, 10 (2), 119-137. doi:10.5455/jeas.2023110109 Chicago Style Laila Almutairi. "Deep Learning based Frameworks for Real-time Cyber Threat Analysis." Journal of Engineering and Applied Sciences 10 (2023), 119-137. doi:10.5455/jeas.2023110109 MLA (The Modern Language Association) Style Laila Almutairi. "Deep Learning based Frameworks for Real-time Cyber Threat Analysis." Journal of Engineering and Applied Sciences 10.2 (2023), 119-137. Print. doi:10.5455/jeas.2023110109 APA (American Psychological Association) Style Laila Almutairi (2023) Deep Learning based Frameworks for Real-time Cyber Threat Analysis. Journal of Engineering and Applied Sciences, 10 (2), 119-137. doi:10.5455/jeas.2023110109 |
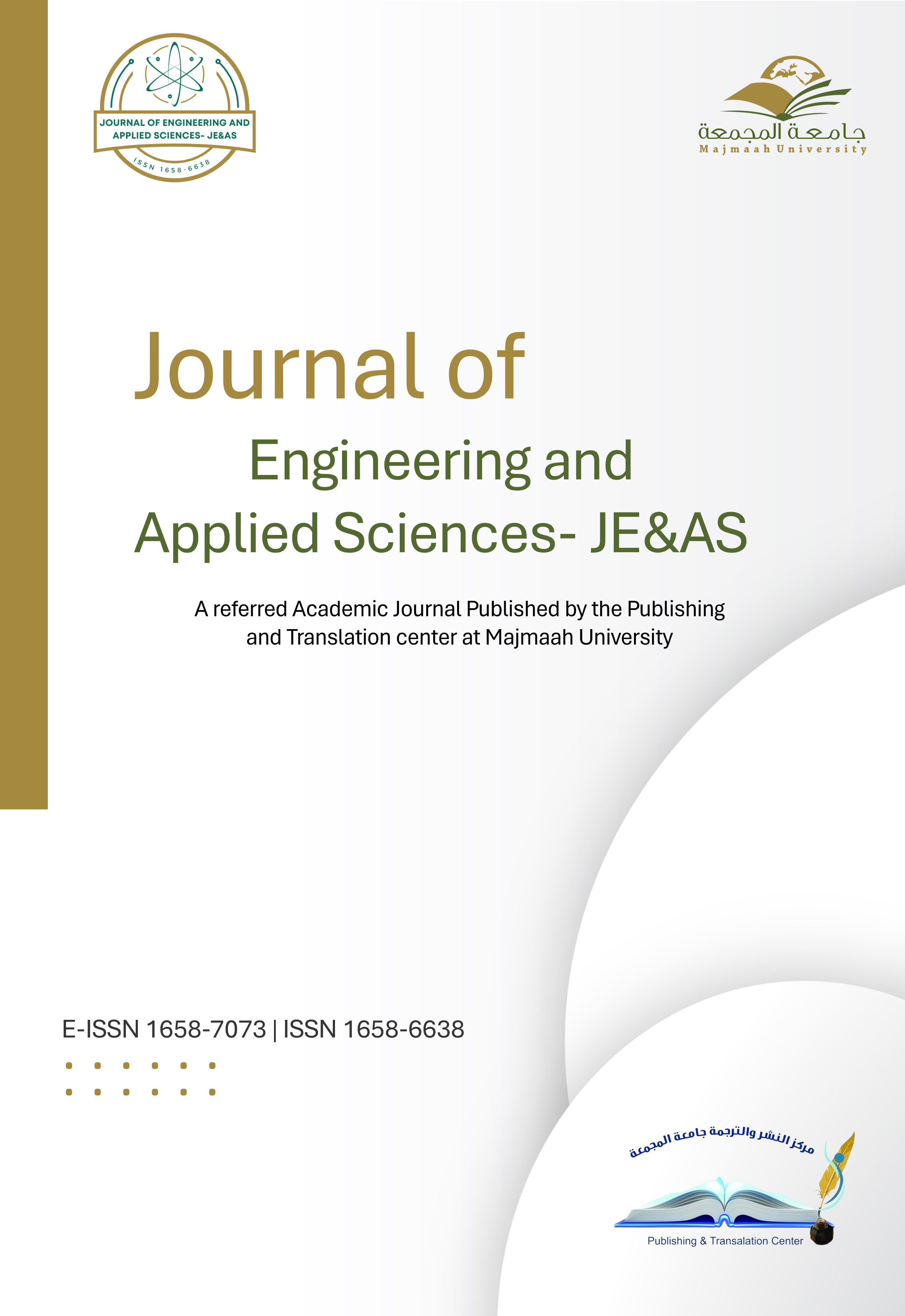


- Author Login
- Reviewer Login
- About Publisher
- Editorial Review Policy
- Peer Review Policy
- Editorial & Peer Review Process
- Author's Rights and Obligations
- Publication Ethics and Publication Malpractice Statement
- Conflict of Interest Policy
- Plagiarism Policy
- Protection of Research Participants (Statement On Human And Animal Rights)
- Privacy Policy
- Self-Archiving Policies
- Statement of Informed Consent
- License Information
- Copyright Information
- ISSN: 1658-6638 (print)
- ISSN: 1658-7073 (electronic)